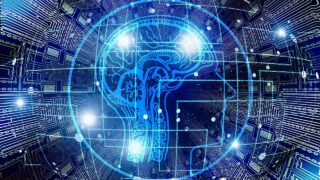
Protected: Calculation of marginal probability distributions – Probability Propagation Method
Compute the probability distribution around graphical models in probabilistic generative models used in digital transformation, artificial intelligence , and machine learning tasks, such as Bayesian estimation, using probability propagation methods