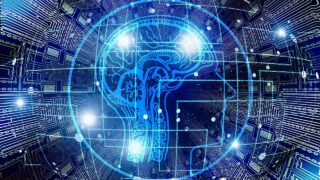
Protected: Maximum Propagation Method for Calculating MAP Assignments in Graphical Models
Estimating the maximized state of probability values (MAP assignment) with the maximum propagation method in probabilistic generative models used in digital transformation, artificial intelligence, and machine learningtasks (TRW maximum propagation method, STA condition, maximum propagation method on a factor graph with cycles, maximum propagation on a tree graph, MAP estimation by message propagation)