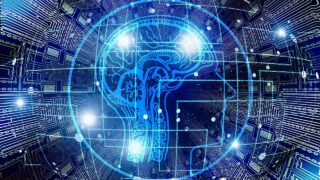
Protected: Reinforcement Learning with Function Approximation (2) – Function Approximation of Value Functions (For Online Learning)
Theory of function approximation online methods gradient TD learning, least-squares based least-squares TD learning (LSTD), GTD2)for reinforcement learning with a huge number of states used in digital transformation , artificial intelligence , and machine learning tasks, and regularization with LASSO.