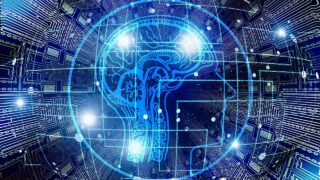
Protected: Mathematical Properties and Optimization of Sparse Machine Learning with Atomic Norm
Mathematical properties and optimization of sparse machine learning with atomic norm for digital transformation, artificial intelligence, and machine learning tasks L∞ norm, dual problem, robust principal component analysis, foreground image extraction, low-rank matrix, sparse matrix, Lagrange multipliers, auxiliary variables, augmented Lagrangian functions, indicator functions, spectral norm, robust principal component analysis, Frank-Wolfe method, alternating multiplier method in duals, L1 norm constrained squared regression problem, regularization parameter, empirical error, curvature parameter, atomic norm, prox operator, convex hull, norm equivalence, dual norm