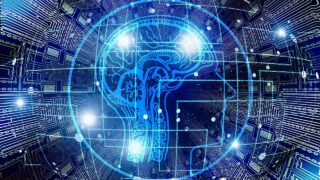
Protected: Equivalence of neural networks (deep learning) and Gaussian processes
On the equivalence of Gaussian processes and neural networks, an applied model of stochastic generative models used in digital transformation, artificial intelligence, and machine learning tasks from Neal's paper