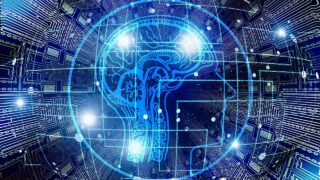
Statistical Feature Extraction(PCA,LDA,PCS,CCA)
Statistical feature extraction (Principal Component Analysis (PCA), Whitening, Fisher Linear Discriminant Analysis (LDA), Canonical Correlation Analysis (CCA), Partial Least Squares (PLS)) for robust local feature extraction in image recognition used in digital transformation (DX) and artificial intelligence (AI).