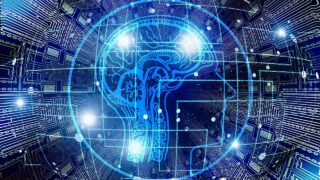
Protected: Regret Analysis for Stochastic Banded Problems
Regret analysis for stochastic banded problems utilized in digital transformation, artificial intelligence, and machine learning tasks (sum of equal sequences, gamma function, Thompson extraction, beta distribution, hem probability, Mills ratio, partial integration, posterior sample, conjugate prior distribution, Bernoulli distribution, cumulative distribution function, expected value, DMED measure, UCB measure, Chernoff-Hefding inequality, likelihood, upper bound, lower bound, UCB score, arms)