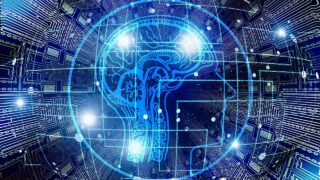
Protected: Stochastic Generative Models and Gaussian Processes(3) Representation of Probability Distributions
Stochastic generative models utilized in digital transformation, artificial intelligence, and machine learning tasks and representation of probability distributions in samples as a basis for Gaussian processes ,weighted sampling, kernel density estimation, distribution estimation using neural nets