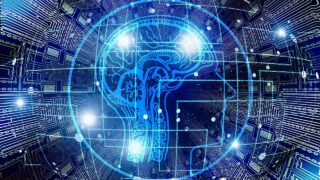
Protected: Overview of nu-Support Vector Machines by Statistical Mathematics Theory
Overview of nu-support vector machines by statistical mathematics theory utilized in digital transformation, artificial intelligence, and machine learning tasks (kernel functions, boundedness, empirical margin discriminant error, models without bias terms, reproducing nuclear Hilbert spaces, prediction discriminant error, uniform bounds Statistical Consistency, C-Support Vector Machines, Correspondence, Statistical Model Degrees of Freedom, Dual Problem, Gradient Descent, Minimum Distance Problem, Discriminant Bounds, Geometric Interpretation, Binary Discriminant, Experience Margin Discriminant Error, Experience Discriminant Error, Regularization Parameter, Minimax Theorem, Gram Matrix, Lagrangian Function).