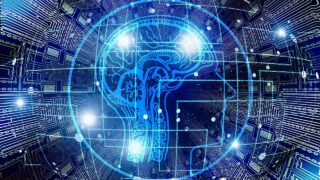
Protected: Variational Bayesian Learning Framework and Algorithms
Overview of variational Bayesian learning and algorithms (variational Bayesian learning, empirical variational Bayesian learning) for approximate computation of complex models in stochastic generative models used in digital transformation, artificial intelligence, and machine learning tasks.