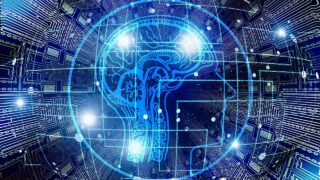
Protected: Correlation, Causation and Relational Structure (2) Backdoor Criteria
Actual backdoor criteria for narrowing down variables to observe intervention effects in causal inference for digital transformation , artificial intelligence, and machine learning tasks