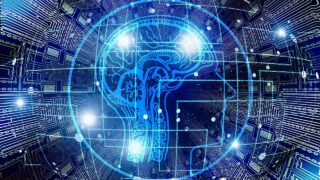
Protected: Research Trends in Deep Reinforcement Learning: Meta-Learning and Transfer Learning, Intrinsic Motivation and Curriculum Learning
Research trends in deep reinforcement learning for digital transformation, artificial intelligence, and machine learning tasks: meta-learning and transfer learning, intrinsic motivation and curriculum learning automatic curriculum generation, automatic task decomposition, task difficulty adjustment, intrinsic reward, robot domain transformation, robot domain transformation, simulator to simulator transfer learning, BERT, Metric/Representation Base, Memory/Knowledge Base, active learning, meta-learning, and robot domain transformation) Robot domain transformation, transfer learning from simulators, BERT, Model-Agnostic Meta-Learning, Active Learning, Metric/Representation Base, Memory/Knowledge Base, Weigh Base, and Learning to Optimize