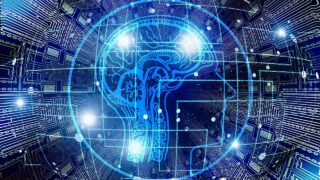
Protected: Statistical Mathematical Theory for Boosting
Statistical and mathematical theory boosting generalized linear model, modified Newton method, log likelihood, weighted least squares method, boosting, coordinate descent method, iteratively weighted least squares method, iteratively reweighted least squares method, IRLS method, weighted empirical discriminant error, parameter update law, Hessian matrix, corrected Newton method, Newton method, Newton method, iteratively reweighted least squares method, IRLS method) used for digital transformation, artificial intelligence, machine learning tasks. iteratively reweighted least square method, IRLS method, weighted empirical discriminant error, parameter update law, Hessian matrix, corrected Newton method, modified Newton method, Newton method, Newton method, link function, logistic loss, logistic loss, boosting algorithm, logit boost, exponential loss, convex margin loss, adaboost, weak hypothesis, empirical margin loss, nonlinear optimization